What is the “AI winter” and when to expect it
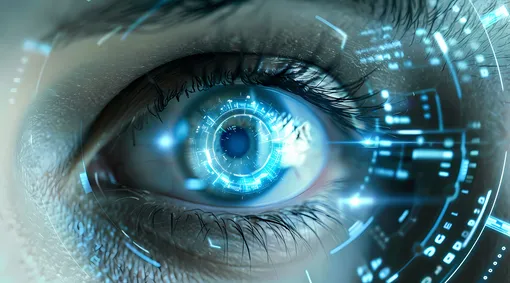
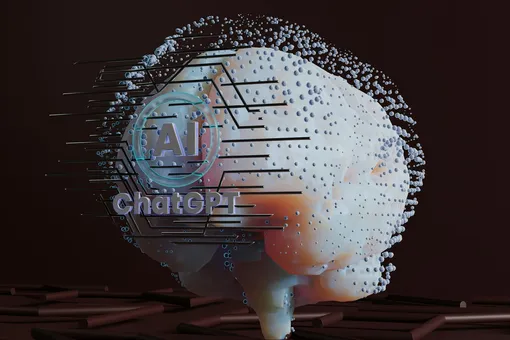
Where does the term “AI winter” come from?
The term “AI winter” was first coined in 1984 at a meeting of the American Association for Artificial Intelligence (AAAI). Researchers Roger Schank and Marvin Minsky used it to describe periods of sharp decline in interest in AI technologies, caused by inflated expectations and subsequent disappointment with the technology. During such periods, investment plummets, media and public attention wanes, and the pace of technological development slows significantly.
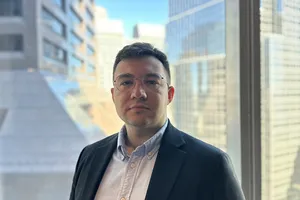
What happened in the previous two “winters”?
The first AI winter began in 1974 after a pessimistic report by British mathematician James Lighthill, who claimed that real progress in AI was limited to solving primitive problems. This caused a sharp reduction in funding in the field in the UK and the US, and research virtually stopped until 1980.
The second winter came in the late 1980s. Fueled by enthusiasm for “expert systems” (a special class of computer programs) that could solve problems in narrow areas, governments and companies invested billions of dollars in the field. But it soon became clear that such systems were too expensive and impractical, and companies switched to more efficient personal computers from Apple and IBM. Disappointment led to a further decline in investment, and AI development virtually froze until the late 1990s.
Where are we now?
Today we are again witnessing a boom in artificial intelligence, especially after the emergence of models ChatGPT, Claude, Gemini and others. It can be said that at this stage of the industry’s development, we are at the very beginning of “spring”. However, some experts are already voicing concerns that another “winter” may be just around the corner. Let’s figure out why they think so.
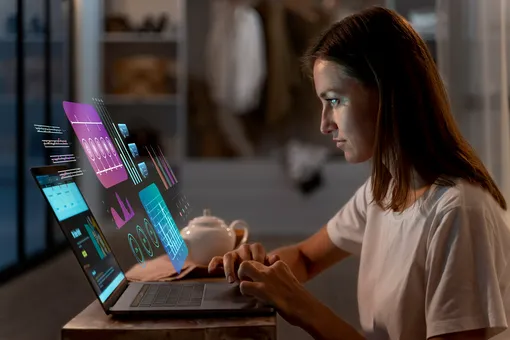
What’s indicative of the next ‘AI winter’?
Among the main reasons for a possible future “cooling” are:
- Inflated expectations. The implementation of AI technologies often does not meet the economic expectations of companies. Most projects require huge financial investments, but do not have a colossal, and most importantly, rapid economic effect. And 90% of AI startups generally fail completely.
- High Computing Costs: Modern AI models require massive computing resources and expensive data centers, which limits scalability. Gartner predicts that 40% of existing data centers for AI workloads will face power constraints by 2027.
- Regulatory risks. Legislative regulation, especially in Europe and the US, is becoming more stringent, imposing restrictions on the use of neural networks and access to data. For example, last year the European Union approved the AI Act, the first comprehensive law on AI. It limits the use of neural networks, especially in sensitive areas (healthcare, education, justice, public administration).
- Environmental issues. Neural networks consume a huge amount of electricity, which increases the environmental pressure on the industry. Comparing data on the amount of CO2 emissions from data centers and aviation, we can conclude that in 5-10 years, AI will cause more harm to the environment than even the global aviation industry.
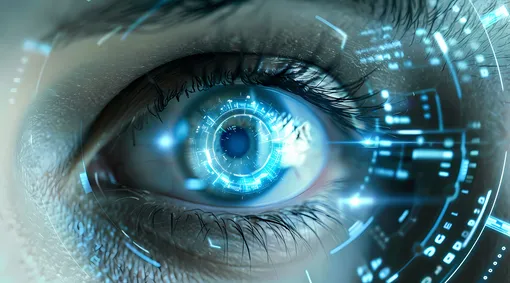
Breakthroughs and Limitations
AI has made huge strides in word processing, but in other areas, progress is limited. Video generation, for example, remains imperfect—even cutting-edge models like OpenAI’s Sora still have artifacts including odd facial expressions and incorrect anatomy. Robotics lacks versatility and fluidity of movement, despite advances from Boston Dynamics and Figure AI. In music generation, products like Suno and Udio show potential but have yet to achieve widespread commercialization.
Therefore, in the coming years we will not see an “AI winter”, but a period when the main achievements of neural networks will be actively implemented in industries and people’s everyday lives. There will be truly useful tools that can significantly simplify human work and increase efficiency in many areas. However, there may be a period of cooling of the market in text technologies, when we will no longer have significant technological breakthroughs here, and the focus will shift to other areas, such as image generation and the development of robotics. But in general, this will not affect the AI industry.
When will the next “winter” come?
A full-fledged “AI winter” may not occur earlier than in 15-30 years , if current architectures exhaust themselves and new solutions prove ineffective. However, the potential remains enormous. In Russia, according to industry analysts, less than half of large companies have implemented AI in their processes. This means that even in conditions of partial cooling of interest, technologies will actively develop and bring benefits.
In the near future, we can expect a “summer/fall” season that will not be a recession, but a transition to a more mature phase of AI development, with an emphasis on the practical value and sustainability of solutions.